Why poor data quality can become a cost hazard
November 09, 2023
As data becomes the new fuel for business around the world, the quality of the data becomes more relevant. There is no doubt that Raw data is surely valuable, but still, a system is required to refine it and maintain its quality.
Weak data quality can take a significant toll on businesses, organisations, and individuals alike. Inaccurate, incomplete, or inconsistent data can lead to flawed decision-making, operational inefficiencies, and compromised customer experiences. The cost of weak data quality extends beyond financial losses, permeating through reputational damage, missed opportunities, and compliance breaches.
There are many ways to measure the value. According to Forbes, studies have shown that businesses with lower data quality can lose around $3.1 trillion in the U.S. alone, or 20% of their value. But a million-dollar question is: why does poor data quality cost a lot of money?
Poor data quality wastes everyone’s time
Unmanaged data is a massive timewaster in every department, including core people like data scientists, data engineers, and data consumers like salespeople. According to Gartner, poor data quality affects labour productivity by up to 20%.
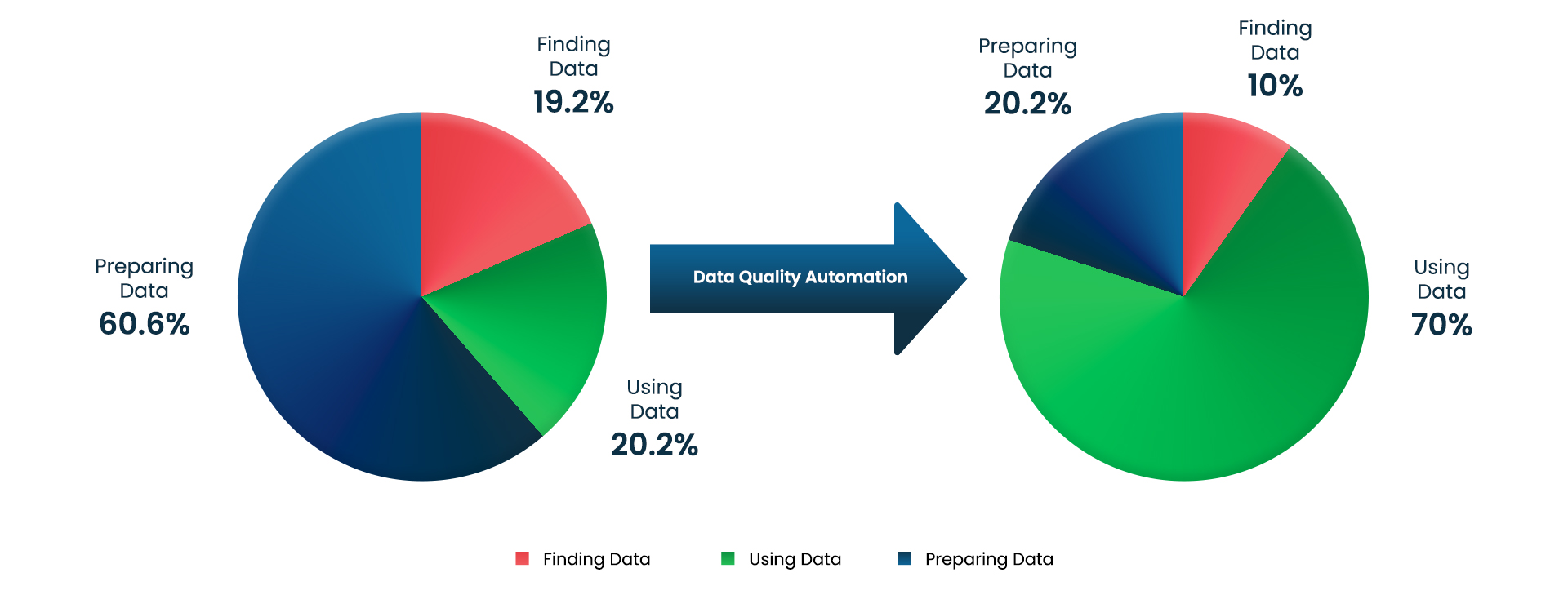
The diagram above depicts the average difference between each role once a robust data quality automation tool is in place. So, one of the most common problems among companies is that, once their data sets arise, the data gets recopied and distributed among other interconnected teams. Therefore, one of the biggest pain points for any data-driven organisation is getting the right data to the right people at the right time.
With data quality automation, organisations can work on the data, which is ready to be utilised upon arrival. Decision-makers can make routine decisions without waiting for their data to become verified and actionable.
Poor data quality leads to poor analytics
Poor data quality is a primary obstacle to effective and accurate analytics. The phrase "garbage in, garbage out" is often used to describe the direct impact of poor data quality on analytical outcomes. Here's how poor data quality leads to poor analytics:
- Inaccurate Insights: If the data used for analytics is inaccurate, incomplete, or outdated, the insights generated from the analysis will be equally flawed. Incorrect data can lead to erroneous conclusions and misinformed decisions.
- Bias and Misrepresentation: Poor data quality can introduce biases into the analysis, skewing the results and leading to misrepresentations of the actual situation. Biased data can perpetuate unfair or discriminatory outcomes, affecting various aspects of an organization's operations.
- Unreliable Predictions: Predictive analytics heavily relies on historical data to forecast future trends and outcomes. If historical data is of poor quality, the predictive models will be less reliable and may fail to accurately forecast future events.
- Inconsistent Reports: When data quality is inconsistent, it becomes challenging to create standardized and reliable reports. Decision-makers might receive conflicting reports from different sources, making it difficult to draw meaningful conclusions.
- Increased Uncertainty: Poor data quality introduces uncertainty and doubt into the decision-making process. Stakeholders may lose confidence in the analytics and become hesitant to base critical decisions on the insights derived from the data.
- Wasted Resources: Data analytics requires time, effort, and resources. If the data used for analysis is of poor quality, all the resources invested in the analytics process may go to waste, yielding minimal value or potentially misleading results.
Poor Data Visualization: Data visualization is a powerful tool for presenting complex information in a digestible manner. However, poor data quality can lead to misleading visualizations that convey inaccurate information, hindering effective communication.
Poor data quality ruins customer experience
poor data quality can indeed ruin the customer experience. Customer experience is a critical factor for any business's success, and data plays a crucial role in understanding, personalizing, and improving that experience. When data quality is compromised, it can have several negative impacts on the customer experience:
- Inaccurate Personalization: Data quality issues can lead to incorrect customer profiles and preferences. As a result, businesses may struggle to provide personalized experiences, leading to irrelevant recommendations and offers that do not resonate with the customers.
- Misguided Customer Communication: Poor data quality can result in inaccurate contact details or communication preferences. This can lead to customers receiving irrelevant or untimely messages, causing frustration and potentially pushing them away.
- Customer Service Failures: In customer service interactions, accurate and up-to-date data is essential for efficient issue resolution and personalized support. When agents have incomplete or incorrect customer information, it can lead to longer resolution times and unsatisfactory customer service experiences.
- Inconsistent Customer Journey: Inconsistent data across various touchpoints can disrupt the customer journey. Customers may need to repeat information or face different experiences depending on the channel they use, leading to a fragmented and frustrating journey.
- Lost Trust and Credibility: When customers encounter errors in their interactions with a company, it erodes their trust and confidence in the brand. Repeated instances of poor data quality can lead customers to question the organization's reliability and credibility.
- Missed Opportunities to Delight Customers: Accurate data enables businesses to identify opportunities to surprise and delight customers. However, poor data quality may prevent businesses from recognizing such moments and providing exceptional experiences.
- Impact on Customer Loyalty and Retention: A negative customer experience resulting from poor data quality can lead to customer churn. Dissatisfied customers are more likely to seek alternatives and switch to competitors who can better meet their needs.
Reputation Damage: Poor data quality issues can become public, especially in cases of data breaches or improper data handling. Such incidents can severely damage a company's reputation and brand image, deterring potential.
Don’t lose valuable resources, time, and money
Ultimately, poor data quality leads to lost revenue, missed opportunities, and flawed situational analysis. To avoid all these, organisations have to prioritise data quality management while investing in data quality initiatives as soon as possible.
Investing in ways to streamline data collection and processing can pay off in a huge way. When your data is fully automated and processed properly, organisations can find the right direction and gain momentum with enhanced revenue streams.
Start with Systems Limited - Keep your data clean
To operate at its best, organisations need high-quality data; this will help them stay informed, increase efficiency, and maximise overall revenue.
Systems limited data quality solutions will let you leverage your weak data quality estate. Our industry-driven solutions are designed to leverage individual business needs. For more information, visit our data and analytics page.
Quick Link
You may like
Streamlining your workforce: How an employer of record (EoR) benefits both global and local hiring
READ MORE